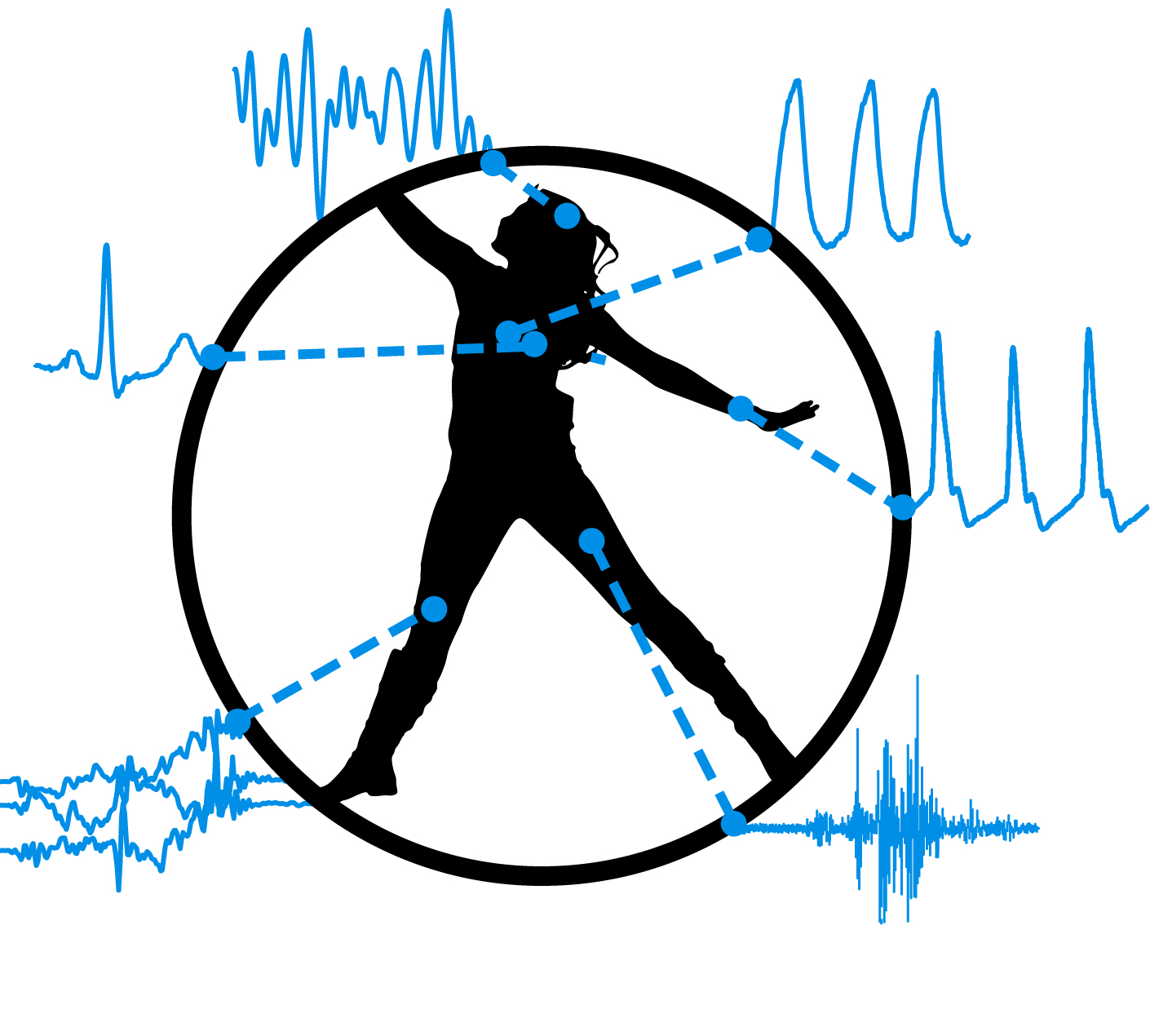
The main goal of our research in this area is the development of new data analysis tools to characterize and interpret the physiological signals that are affected by different disorders. We specifically focus on sleep and epilepsy monitoring. Some active areas of research are multimodal approaches to machine learning, data-centric AI, reducing the number of electrodes that patients need to wear, dealing with artifacts, and transfer learning and domain adaptation methods to reduce the dependency on large labeled datasets and improve generalization. By developing such systems, the aim is to improve the quality of life of patients, as well as the diagnosis, follow-up, and monitoring of different disorders.