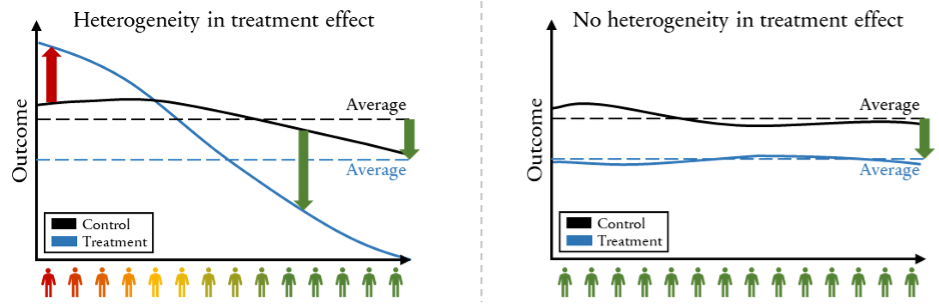
Machine learning can help in modelling these effect using conditional average treatment effect models which use the data of the patients in the different arms to estimate individual treatment effect. As such, the aim is to identify who will benefit from the treatment and who should not be treated. One important methodological limitation is that in order to calculate the true treatment effect for one subject, the outcomes under treatment and control conditions have to be known, which is realistically impossible. This is commonly known as the fundamental problem of causal inference. A big challenge in this research area is to deal with this important limitation and to maximize the information extracted from the different arms in the randomized controlled trials.