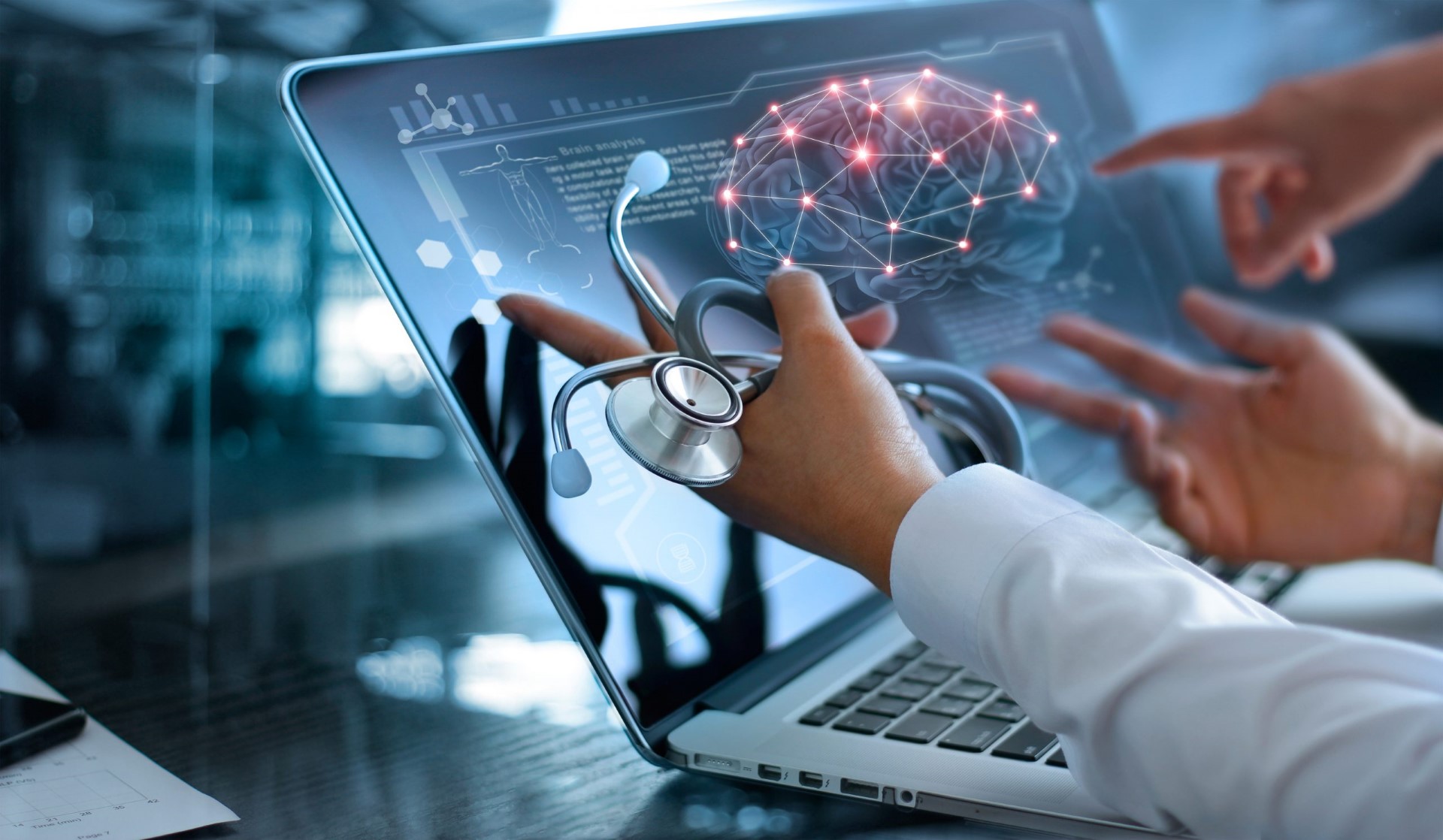
An open source library containing interactive implementations of several popular post-hoc explanation techniques. These allow (medical) end-users to correct explanations which can then be enforced to improve the model significantly.
As machine learning models grow in complexity and find increased application in medical settings, there is a growing demand to comprehend and oversee the decisions made by these models. While various approaches to elucidate black box models already exist, their practical utility often lacks substantial evidence. Within our group, we strive to address this gap by introducing innovative methods that effectively leverage explanations in medical environments. Our goal is to enhance trust in these models and elevate their overall quality through meaningful application of explanatory techniques.
An open source library containing interactive implementations of several popular post-hoc explanation techniques. These allow (medical) end-users to correct explanations which can then be enforced to improve the model significantly.