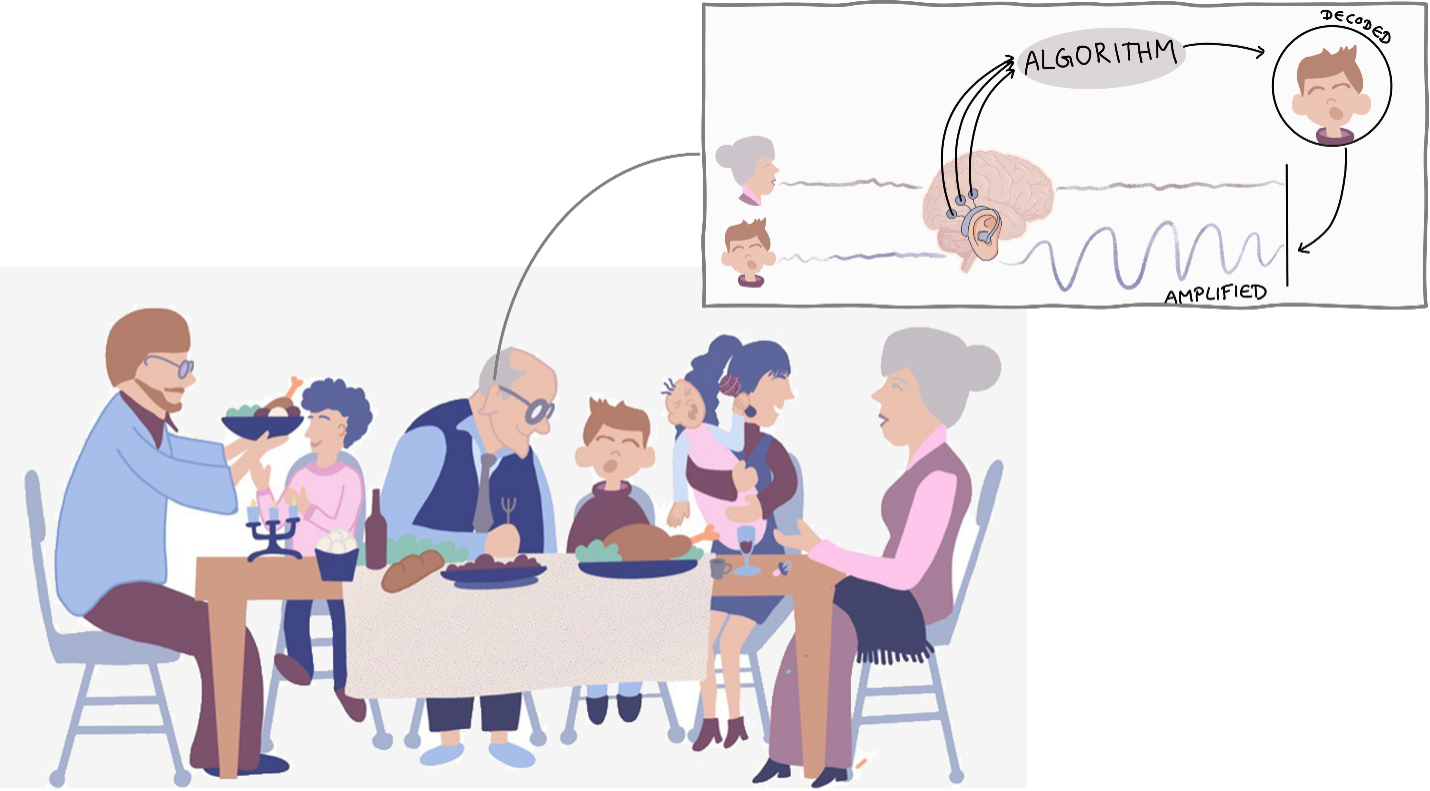
State-of-the-art hearing aids and cochlear implants underperform in a so-called ‘cocktail party’ scenario when multiple persons talk simultaneously (think of a reception or family dinner party). In such situations, the hearing device does not know which speaker the user intends to attend to and, thus, which speaker to enhance and which other ones to suppress. Over the past years, we have developed various algorithms and technology that allow decoding the brain activity of listeners in cocktail party problems to determine which speaker a user is attending to. With this technology, we envisage a cognitively-controlled hearing aid that allows users to steer the hearing aid towards the speech source they want to listen to.